WACV 2024 Workshop on
Rich Media with Generative AI
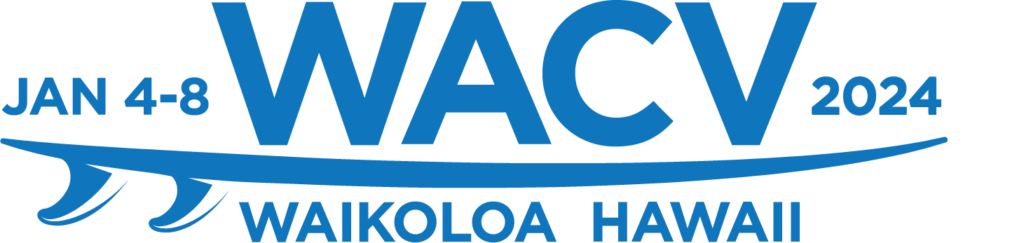
Overview
The goal of this workshop is to showcase the latest developments of generative AI for creating, editing, restoring, and compressing rich media, such as images, videos, audio, neural radiance fields, and 3D scene properties. Rich media are widely used in various domains, such as video games, smartphone photography, visual simulation, AR/VR, medical imaging, and robotics. Generative AI models, such as generative adversial networks (GAN), diffusion models, and large language models (LLM), have enabled remarkable achievements in rich media from both academia research and industrial applications, such as Stable Diffusion, Midjourney, Dream Booth (Google), Dream Booth 3D (Google), Firefly (Adobe), Picasso (NVIDIA), VoiceBox (Meta), DALL-E2 (OpenAI).
This workshop aims to provide a platform for researchers in this field to share their latest work and exchange their ideas. The topics in this workshop include but are not limited to:
- Restoration and enhancement of rich media with generative AI
- Editing and manipulation of rich media with generative AI
- Compression and codec design with generative AI
- Image-based modeling and rendering with generative AI
- Neural radiance fields with generative AI
- Rich media creation and editing with large language models
- Acceleration of generative AI models on edge devices
- Bias and Forgery detection in the era of generative AI
Speaks
Jun-Yan Zhu is an Assistant Professor at CMU’s School of Computer Science. Prior to joining CMU, he was a Research Scientist at Adobe Research and a postdoc at MIT CSAIL. He obtained his Ph.D. from UC Berkeley and B.E. from Tsinghua University. He studies computer vision, computer graphics, and computational photography. His current research focuses on generative models for visual storytelling. He has received the Packard Fellowship, the NSF CAREER Award, the ACM SIGGRAPH Outstanding Doctoral Dissertation Award, and the UC Berkeley EECS David J. Sakrison Memorial Prize for outstanding doctoral research, among other awards.
Chen-Hsuan Lin is a senior research scientist at NVIDIA Research, working on computer vision, computer graphics, and generative AI applications. He is interested in solving problems for 3D content creation, involving 3D reconstruction, neural rendering, generative models, and beyond. His research aims to empower AI systems with 3D visual intelligence: human-level 3D perception and imagination abilities. His research has been recognized with a Best Inventions of 2023 by TIME Magazine.
Xingang Pan is an Assistant Professor with the School of Computer Science and Engineering at Nanyang Technological University, affiliated with MMLab-NTU and S-Lab. Prior to joining NTU, he was a postdoc researcher at Max Planck Institute for Informatics, advised by Prof. Christian Theobalt. He received his Ph.D. degree at MMLab of The Chinese University of Hong Kong in 2021, supervised by Prof. Xiaoou Tang. He obtained his Bachelor’s degree from Tsinghua University in 2016. His research interests include computer vision, machine learning, and computer graphics, with a focus on generative AI and neural rendering.
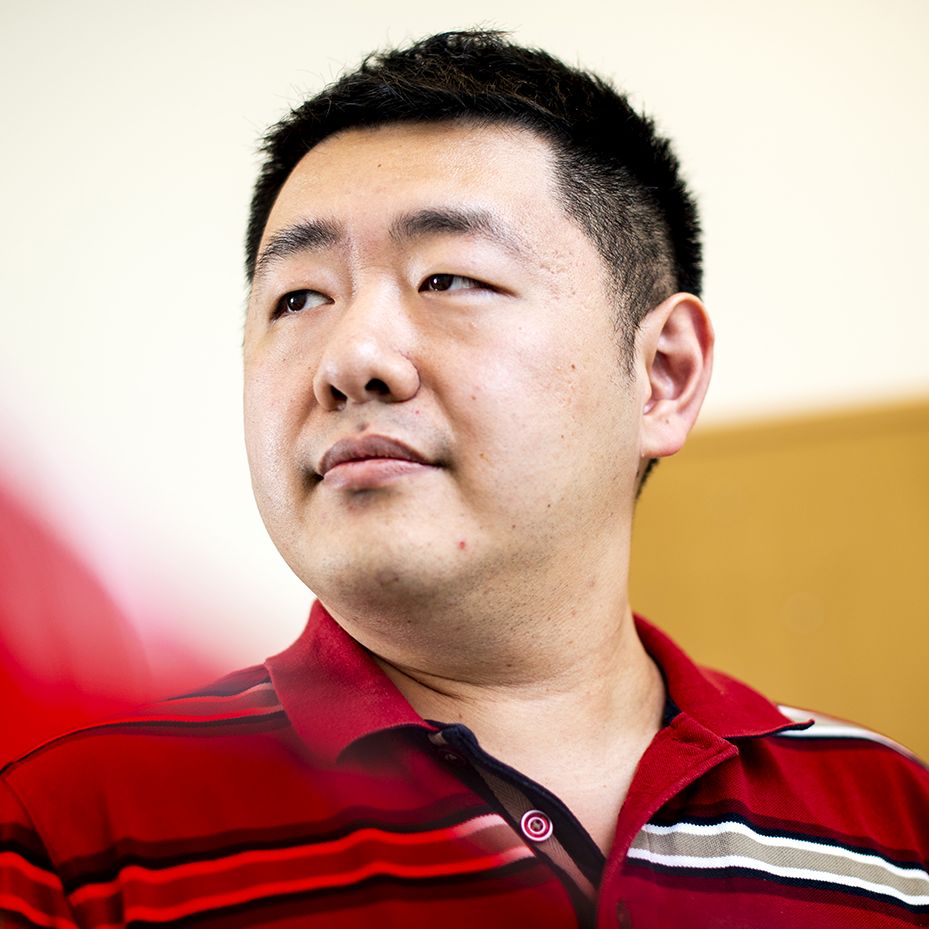
Northeastern Univeristy
Yanzhi Wang is currently an associate professor and faculty fellow at Dept. of ECE at Northeastern University, Boston, MA. He received the B.S. degree from Tsinghua University in 2009, and Ph.D. degree from University of Southern California in 2014. His research interests focus on model compression and platform-specific acceleration of deep learning applications. His work has been published broadly in top conference and journal venues (e.g., DAC, ICCAD, ASPLOS, ISCA, MICRO, HPCA, PLDI, ICS, PACT, ISSCC, AAAI, ICML, NeurIPS, CVPR, ICLR, IJCAI, ECCV, ICDM, ACM MM, FPGA, LCTES, CCS, VLDB, PACT, ICDCS, RTAS, Infocom, C-ACM, JSSC, TComputer, TCAS-I, TCAD, TCAS-I, JSAC, TNNLS, etc.), and has been cited above 16,000 times. He has received six Best Paper and Top Paper Awards, and one Communications of the ACM cover featured article. He has another 13 Best Paper Nominations and four Popular Paper Awards. He has received the U.S. Army Young Investigator Program Award (YIP), IEEE TC-SDM Early Career Award, APSIPA Distinguished Leader Award, Massachusetts Acorn Innovation Award, Martin Essigmann Excellence in Teaching Award, Massachusetts Acorn Innovation Award, Ming Hsieh Scholar Award, and other research awards from Google, MathWorks, etc. He has received 26 federal grants from NSF, DARPA, IARPA, ARO, ARFL/AFOSR, Dept. of Homeland Security, etc. He has participated in a total of $40M funds with personal share $8.5M. 11 of his academic descendants become tenure track faculty at Univ. of Connecticut, Clemson University, Chongqing University, University of Georgia, Beijing University of Technology, University of Texas San Antonio, and Cleveland State University. They have secured around $5M personal share in funds.
Schedule
Hover over the titles to view the abstracts of each talk.
Title | Speaker | Slides | Video | Time |
---|---|---|---|---|
Opening Remarks | Zhixiang Wang | Link | ||
Data Ownership in Generative Models
Large-scale generative visual models, such as DALL·E2 and Stable Diffusion, have made content creation as little effort as writing a short text description. However, these models are typically trained on an enormous amount of Internet data, often containing copyrighted material, licensed images, and personal photos. How can we remove these images if creators decide to opt-out? How can we properly compensate them if they choose to opt in? In this talk, I will first describe an efficient method for removing copyrighted materials, artistic styles of living artists, and memorized images from pretrained text-to-image diffusion models. I will then discuss our data attribution algorithm for assessing the influence of each training image for a generated sample. Collectively, we aim to enable creators to retain control over the ownership of training images. |
Jun-Yan Zhu | Link | -- | |
Diffusion Models for 3D Asset Generation
3D digital content has been in high demand for a variety of applications, including gaming, entertainment, architecture, and robotics simulation. However, creating such 3D content requires professional 3D modeling expertise with a significant amount of time and effort. In this talk, I will talk about recent advances on automating high-quality 3D digital content creation from text prompts. I will also cover Magic3D, which can generate high-resolution 3D mesh models from input text descriptions, as well as our recent efforts on 3D generative AI with NVIDIA Picasso. With these text-to-3D approaches, we aim to democratize and turbocharge 3D content creation for all, from novices to expert 3D artists. |
Chen-Hsuan Lin | |||
Coffee Break | ||||
Harnessing Deep Generative Models for Point-Dragging Manipulation and Image Morphing
In this talk, I will first present DragGAN, an interactive point-dragging image manipulation method. Unlike previous works that gain controllability of GANs via manually annotated training data or a prior 3D model, DragGAN allows users to "drag" any points of the image to precisely reach target points, thus manipulating the pose, shape, expression, and layout of diverse categories such as animals, cars, humans, landscapes, etc. While DragGAN produces continuous animations, such effects are hard to obtain on diffusion models. So I will then introduce how we address this limitation with DiffMorpher, a method enabling smooth and natural image morphing based on diffusion models. |
Xingang Pan | Link | ||
GPT and Stable Diffusion on the Mobile: Towards Ultimate Efficiency in Deep Learning Acceleration
Mobile and embedded computing devices have become key carriers of deep learning to facilitate the widespread use of machine intelligence. However, there is a widely recognized challenge to achieve real-time DNN inference on edge devices, due to the limited computation/storage resources on such devices. Model compression of DNNs, including weight pruning and weight quantization, has been investigated to overcome this challenge. However, current work on DNN compression suffers from the limitation that accuracy and hardware performance are somewhat conflicting goals difficult to satisfy simultaneously. We present our recent work Compression-Compilation Codesign, to overcome this limitation towards the best possible DNN acceleration on edge devices. The neural network model is optimized in a hand-in-hand manner with compiler-level code generation, achieving the best possible hardware performance while maintaining zero accuracy loss, which is beyond the capability of prior work. We are able to achieve real-time on-device execution of a number of DNN tasks, including object detection, pose estimation, activity detection, speech recognition, just using an off-the-shelf mobile device, with up to 180 X speedup compared with prior work. Recently, for the first time, we enable large-scale language and AIGC models such as GPT and Stable Diffusion on mobile devices. We will also introduce our breakthrough in digital avatar, stable diffusion for video generation, and interaction systems. Last we will introduce our recent breakthrough of superconducting logic based neural network acceleration that achieves 10^6 times energy efficiency gain compared with state-of-the-art solutions, achieving the quantum limit in computing. |
Yanzhi Wang |